Smart Roads Guard: Obstacle Detection and Accident Avoidance System
DOI:
https://doi.org/10.26438/ijsrcse.v13i2.631Keywords:
Object detection, YOLO, Accidents, Road, Obstacle, Sustainability, Artificial intelligence, SafetyAbstract
Road accidents continue to remain a serious issue globally, resulting in injuries and deaths. The Smart Roads Guard system aims to curb the problem with its AI-powered obstacle detection and alerting system. It analyzes footage from roadside cameras to detect dangers and activates the flashlights installed along the road at a sufficient distance and time according to the area where the system is used, which can reach up to one kilometer. This technique enables early real-time detection and allows drivers ample opportunity to alter their speed or change lanes to prevent collisions. To maintain functionality in poor visibility conditions, fog lights are positioned above the cameras on lampposts, which increases detection capabilities during fog conditions and reduces costs. A web-based control platform augments the system by allowing stakeholders such as traffic authorities, universities, private firms, or even residents to monitor and receive real-time alerts to enable a swift countermeasure to road dangers. The system is capable of integration with radar; however, this version is limited to camera-based detection due to budgetary constraints. It utilizes solar energy to improve sustainability, maintaining operation even during bad weather. The Smart Roads Guard is particularly appropriate for developed nations and Gulf regions, where sophisticated infrastructure and weather-related issues necessitate creative and dependable traffic safety solutions. However, it also offers significant value for underserved or uncovered areas lacking traditional traffic monitoring systems. By training the AI model on obstacle images using YOLOv8, we achieved 89% accuracy. The Smart Roads Guard system, which represents a significant advancement in intelligent transportation systems and is easy to implement with low cost, will make road networks safer, smarter, and more sustainable.
References
World Health Organization, “Global Status Report on Road Safety 2023,” First Edition, World Health Organization Publisher, Switzerland, pp.1-400, 2023. ISBN: 9789240086517.
Saudi Vision 2030, “National Transformation Program,” First Edition, Saudi Government Publisher, Saudi Arabia, pp.1-100, 2016.
S.K. Sanil, K.S. Balamurugan, S.K. Subramani, “An Insight into Crash Avoidance and Overtaking Advice Systems for Autonomous Vehicles: A Comprehensive Review,” Engineering Applications of Artificial Intelligence, Vol.103, Issue. -, pp.1-20, 2021. DOI: 10.1016/j.engappai.2021.104288.
M.A. Hadi, M.A. Hossain, M.A. Rahman, “Improved VIDAR and Machine Learning-Based Road Obstacle Detection for Self-Driving Systems,” Journal of Traffic and Transportation Engineering (English Edition), Vol.10, No.1, pp.103-117, 2023. DOI: 10.1016/j.jtte.2023.01.001.
X. Li, Y. Zhang, J. Wang, “Real-Time Pedestrian Detection Using Deep Learning for Intelligent Transportation Systems,” IEEE Transactions on Intelligent Transportation Systems, Vol.23, No.1, pp.178-190, 2022. DOI: 10.1109/TITS.2021.3114044.
A.K. Maurya, D.P. Dogra, “Obs-tackle: An Obstacle Detection System to Assist Navigation of Visually Impaired,” Machine Vision and Applications, Vol.34, No.1, p.8, 2023. DOI: 10.1007/s00138-023-01499-8.
G. Arvanitis, N. Stagakis, E.I. Zacharaki, K. Moustakas, “Cooperative Saliency-Based Obstacle Detection and AR Rendering for Increased Situational Awareness,” arXiv Preprint, arXiv:2302.00916, 2023.
J. Redmon, A. Farhadi, “YOLOv3: An Incremental Improvement,” arXiv Preprint, arXiv:1804.02767, 2018.
A. Rezaei, H. Mousavi, S. Sadri, “A Comprehensive Review of YOLO Architectures in Computer Vision: From YOLOv1 to YOLOv8 and YOLO-NAS,” arXiv Preprint, arXiv:2304.00501, 2023.
X. Li, J. Wang, H. Zhang, “Dynamic Obstacle Avoidance Model of Autonomous Driving with Deep Reinforcement Learning,” Alexandria Engineering Journal, Vol.2024, No.8779, pp.1-12, 2024. DOI: 10.1016/j.aej.2024.04.005.
M. Zhao, L. Yu, R. Wang, “Deep Learning for Autonomous Driving Systems,” Computer Engineering and Science, Vol.2024, No.83, pp.1-10, 2024.
S.K. Gupta, A. Verma, P. Kumar, “YOLO-Fusion and Internet of Things: Advancing Object Detection in Autonomous Driving,” Alexandria Engineering Journal, Vol.2024, No.10263, pp.1-10, 2024.
G. Karuna, R.P.R. Kumar, V.T.S. Sai, J. Abhishek, M. Shashikanth, B. Kashyap, “Motorcycle Crash Detection and Alert System Using IoT,” E3S Web of Conferences, Vol.391, p.01145, 2023. DOI: 10.1051/e3sconf/202339101145.
W. Zhao, et al., “Developing a New Integrated Advanced Driver Assistance System in a Connected Vehicle Environment,” Expert Systems with Applications, Vol.238, p.121733, 2023. DOI: 10.1016/j.eswa.2023.121733.
H.M. Al-Ahmadi, “Analysis of Traffic Accidents in Saudi Arabia: Safety Effectiveness Evaluation of SAHER Enforcement System,” Arabian Journal for Science and Engineering, Vol.-, No.-, pp.1-15, 2022. DOI: 10.1007/s13369-022-07473-2.
AAA Foundation, “New AAA Foundation Study: 200,000 Crashes Caused by Road Debris,” First Edition, AAA Foundation Publisher, USA, pp.1-20, 2016.
Z.-Q. Zhao, P. Zheng, S.-T. Xu, X. Wu, “Object Detection With Deep Learning: A Review,” IEEE Transactions on Neural Networks and Learning Systems, Vol.30, No.11, pp.3212-3232, 2019. DOI: 10.1109/TNNLS.2018.2876865.
R. Kaur, S. Singh, “A Comprehensive Review of Object Detection with Deep Learning,” Digital Signal Processing, Vol.132, p.103812, 2023. DOI: 10.1016/j.dsp.2022.103812.
A. Razi, et al., “Deep Learning Serves Traffic Safety Analysis: A Forward‐Looking Review,” IET Intelligent Transport Systems, Vol.17, No.1, pp.22-71, 2022. DOI: 10.1049/itr2.12257.
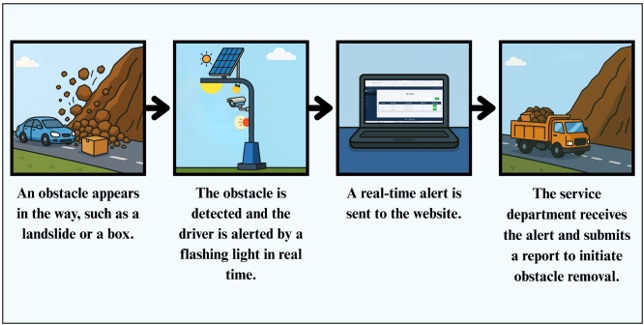
Downloads
Published
How to Cite
Issue
Section
License
Copyright (c) 2025 Dalia El Gezawi, Raghed Aljassim, Batool Alsaffar, Hajer Alsaleh, Zainab Alfandi, Yasmin Alhaji

This work is licensed under a Creative Commons Attribution 4.0 International License.
Authors contributing to this journal agree to publish their articles under the Creative Commons Attribution 4.0 International License, allowing third parties to share their work (copy, distribute, transmit) and to adapt it, under the condition that the authors are given credit and that in the event of reuse or distribution, the terms of this license are made clear.