Comparison of NAIVE, SARIMA, and SARIMAX Models in Short-Term and Long-Term Forecasting of Google Search Trends
DOI:
https://doi.org/10.26438/ijsrcse.v13i2.665Keywords:
Google Search Trends, Forecasting Methods, Naïve Method, SARIMA, SARIMAX, Time Series AnalysisAbstract
This study examines forecasting methods—Naïve, SARIMA, and SARIMAX—to enhance the accuracy of Google search trend predictions in the fitness industry, specifically for the keyword "Gym." The Naïve method serves as a baseline comparison, SARIMA incorporates seasonal, autoregressive, and moving average components for improved trend detection, while SARIMAX extends SARIMA by integrating exogenous variables. Historical Google search data from 2005 to 2015 is used for model evaluation, with performance assessed using MAE, RMSE, and MAPE metrics. The findings indicate that SARIMAX, which accounts for external influences, provides the highest accuracy. Additionally, short-term models exhibit greater responsiveness to seasonal variations. This study offers insights into the strengths and limitations of each method, assisting practitioners in selecting the most suitable approach for improving search trend forecasting and supporting data-driven decision-making in the fitness industry.
References
I. Majid, “Analisis Penerapan Google Custom Search API pada Sistem Pencarian Data,” Journal of Computer Information Systems (J-CIS), Vol.1, pp.29-35, 2021.
L. H. Hasibuan, S. Musthofa, D. M. Putri, M. Jannah, “Comparison of Seasonal Time Series Forecasting Using SARIMA and Holt Winter’s Exponential Smoothing (Case Study: West Sumatra Export Data),” Barekeng Journal of Mathematics and Its Applications, Vol.17, Issue.3, pp.1773-1784, 2023.
N. Suhermi, Suhartono, R. P. Permata, S. P. Rahayu, “Forecasting the Search Trend of Muslim Clothing in Indonesia on Google Trends Data Using ARIMAX and Neural Network,” in Soft Computing in Data Science, M. W. Berry, B. W. Yap, A. Mohamed, M. Köppen (Eds.), Springer Singapore, pp.272-286, 2019.
W. Anggraeni, L. Aristiani, “Using Google Trend Data in Forecasting Number of Dengue Fever Cases with ARIMAX Method Case Study: Surabaya, Indonesia,” In the Proceedings of the 2016 International Conference on Information & Communication Technology and Systems (ICTS), Indonesia, pp.114-118, 2016.
N. Newton, A. Kurnia, I. M. Sumertajaya, “Analisis Inflasi Menggunakan Data Google Trends dengan Model ARIMAX di DKI Jakarta,” Indonesian Journal of Statistics and Its Applications, Vol.4, Issue.3, pp.545-556, 2020.
V. Shah et al., “Forecasting Maximum Temperature Trends with SARIMAX: A Case Study from Ahmedabad, India,” In the Proceedings of Sustainability Conference, 2024.
M. C. Medeiros, H. F. Pires, “The Proper Use of Google Trends in Forecasting Models,” Departamento de Economia, Pontifícia Universidade Católica do Rio de Janeiro (PUC-Rio), pp.1-25, 2021.
D. S. Priyarsono, S. Magfirotul Laelia, “Studi Penggunaan Data Google Trends: Kasus Peramalan Tingkat Pengangguran Usia Muda,” Bina Ekonomi, Vol.27, Issue.2, pp.100-123, 2023.
N. N. Lingga, A. A. Rohmawati, “Pemodelan dan Peramalan Kedatangan Wisatawan ke Tempat Wisata dengan Google Trends Menggunakan Metode Variasi Kalender ARIMAX,” In the Proceedings of the eProceedings of Engineering, Telkom University, Indonesia, pp.1-5, 2023.
A. D. Riyanto, “Pemanfaatan Google Trends dalam Penentuan Kata Kunci Sebuah Produk untuk Meningkatkan Daya Saing Pelaku Bisnis di Dunia Internet,” In the Proceedings of the 2014 Conference on Internet Business Competitiveness, Indonesia, pp.1-5, 2014.
N. Thakur, S. Karmakar, “Deep Learning Approach Using Long Short Term Memory Technique for Monthly Rainfall Prediction in Chhattisgarh, India,” International Journal of Scientific Research in Computer Science and Engineering, Vol.9, Issue.1, 2021.
R. P. Permata, D. D. Prastyo, Wibawati, “Hybrid Dynamic Harmonic Regression with Calendar Variation for Turkey Short-Term Electricity Load Forecasting,” Procedia Computer Science, Elsevier, pp.25-33, 2022.
A. A. Karim, E. Pardede, S. Mann, “A Model Selection Approach for Time Series Forecasting: Incorporating Google Trends Data in Australian Macro Indicators,” Entropy, Vol.25, Issue.8, 2023.
S. Olukanmi, F. Nelwamondo, “Digital Influenza Surveillance: The Prospects of Google Trends Data for South Africa,” In the Proceedings of the 2020 International Conference on Artificial Intelligence, Big Data, Computing and Data Communication Systems (icABCD), South Africa, pp.1-6, 2020.
T. Boone, R. Ganeshan, R. L. Hicks, N. R. Sanders, “Can Google Trends Improve Your Sales Forecast?,” In the Proceedings of the Production and Operations Management Society Conference, USA, 2018.
I. O. Muraina, O. M. Adesanya, M. A. Agoi, S. O. Abam, “The Necessity of Exploratory Data Analysis: How are preprocessing activities beneficial to Data Analysts and Professional Researchers in Academia?,” International Journal of Scientific Research in Computer Science and Engineering, Vol.11, Issue.3, 2023.
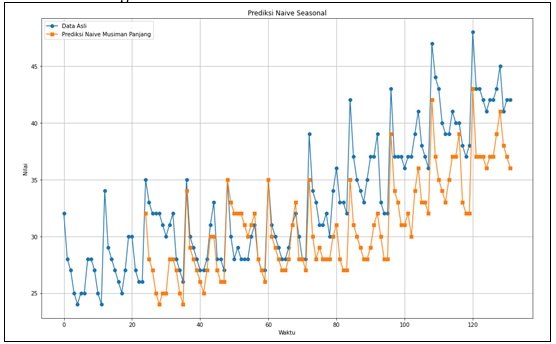
Downloads
Published
How to Cite
Issue
Section
License

This work is licensed under a Creative Commons Attribution 4.0 International License.
Authors contributing to this journal agree to publish their articles under the Creative Commons Attribution 4.0 International License, allowing third parties to share their work (copy, distribute, transmit) and to adapt it, under the condition that the authors are given credit and that in the event of reuse or distribution, the terms of this license are made clear.