A Deep Learning-based Hybrid CNN-LSTM Model for Human Activity Recognition
Keywords:
human activity recognition, CNN-LSTM, hybrid deep learning, multivariate time-series, temporal dependencies, spatial feature extractionAbstract
Human Activity Recognition (HAR) involves identifying and classifying physical activities performed by individuals using data collected from sensors like accelerometers, gyroscopes, or cameras. HAR has broad applications in healthcare, fitness monitoring, and human-computer interaction, where accurate activity recognition can enhance user experiences and provide actionable insights. Despite progress in standalone deep learning models, limitations persist in capturing both spatial and temporal dependencies effectively. To address this, we developed a hybrid deep learning model combining Convolutional Neural Networks (CNNs) and Long Short-Term Memory (LSTM) networks. CNNs were employed to extract spatial features from multivariate time-series sensor data, while LSTMs captured temporal dependencies crucial for accurate classification. The UCI HAR dataset, consisting of six activity labels, was used to benchmark the model. The implementation was carried out in Python, leveraging libraries like TensorFlow and Keras. The proposed hybrid CNN-LSTM model achieved an overall accuracy of 94%, with precision, recall, and F1-scores (macro and weighted averages) also reaching 94%. Individual activity labels recorded F1-scores ranging from 85% to 100%, demonstrating the model`s robustness across diverse activities. These findings validate the effectiveness of the hybrid CNN-LSTM architecture in overcoming the limitations of standalone models. The ability to capture both spatial and temporal patterns in sensor data underscores the model`s potential in advancing HAR applications. This study provides a foundation for future research in refining hybrid approaches, exploring additional datasets, and deploying such models in real-world applications. The results have significant implications for improving healthcare monitoring, fitness tracking, and human-computer interaction systems.
References
A. Ignatov. “Real-time human activity recognition from accelerometer data using Convolutional Neural Networks”. Applied Soft Computing, Issue 62, pp.915-922, 2017. https://doi.org/10.1016/j.asoc.2017.09.027
A. Sharma, P. Singh, C. Madhu, N. Garg and G. Joshi, "Human Activity Recognition with smartphone sensors data using CNN-LSTM," 2022 IEEE Conference on Interdisciplinary Approaches in Technology and Management for Social Innovation (IATMSI), Gwalior, India, 2022, pp.1-6, 2022. doi: 10.1109/IATMSI56455.2022.10119372.
L. Chen, X. Liu, L. Peng, and L. Wu. “Deep learning based multimodal complex human activity recognition using wearable devices.” Applied Intelligence Issue 51, pp.4029–4042, 2021. https://doi.org/10.1007/s10489-020-02005-7
F.J. Ordóñez, and D. Roggen. “Deep Convolutional and LSTM Recurrent Neural Networks for Multimodal Wearable Activity Recognition”. Sensors, Vol.16, Issue 1 pp.1–25, 2016. https://doi.org/10.3390/s16010115
C.A. Ronao, and S. Cho. “Human activity recognition with smartphone sensors using deep learning neural networks”. Expert Systems with Applications, Vol.59, pp.235-244, 2016. https://doi.org/10.1016/j.eswa.2016.04.032
M. Atikuzzaman, T. R. Rahman, E. Wazed, M. P. Hossain and M. Z. Islam, "Human Activity Recognition System from Different Poses with CNN," 2020 2nd International Conference on Sustainable Technologies for Industry 4.0 (STI), Dhaka, Bangladesh, pp.1-5, 2020 doi: 10.1109/STI50764.2020.9350508.
R. Maurya, T. H. Teo, S. H. Chua, H. -C. Chow and I. -C. Wey, "Complex Human Activities Recognition Based on High Performance 1D CNN Model," 2022 IEEE 15th International Symposium on Embedded Multicore/Many-core Systems-on-Chip (MCSoC), Penang, Malaysia, pp.330-336, 2022. doi: 10.1109/MCSoC57363.2022.00059.
Lee, S., Yoon, S.M., & Cho, H. “Human activity recognition from accelerometer data using Convolutional Neural Network”. IEEE International Conference on Big Data and Smart Computing (BigComp), pp.131-134, 2017.
A. Gumaei, M. M. Hassan, A. Alelaiwi and H. Alsalman, "A Hybrid Deep Learning Model for Human Activity Recognition Using Multimodal Body Sensing Data," in IEEE Access, vol. 7, pp.99152-99160, 2019, doi: 10.1109/ACCESS.2019.2927134.
W. Xu, Y. Pang, Y. Yang and Y. Liu, "Human Activity Recognition Based on Convolutional Neural Network," 2018 24th International Conference on Pattern Recognition (ICPR), Beijing, China, pp.165-170, 2018. doi: 10.1109/ICPR.2018.8545435.
Wang, J., Chen, Y., Hao, S., Peng, X., & Hu, L. “Deep learning for sensor-based activity recognition: A survey.” Pattern Recognition Letters, Vol.119, pp.3-11, 2019. https://doi.org/10.1016/j.patrec.2018.02.010
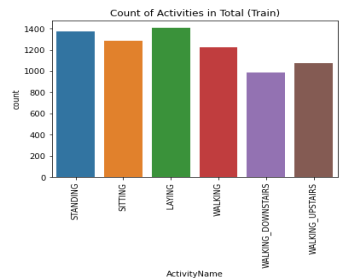
Downloads
Published
How to Cite
Issue
Section
License

This work is licensed under a Creative Commons Attribution 4.0 International License.
Authors contributing to this journal agree to publish their articles under the Creative Commons Attribution 4.0 International License, allowing third parties to share their work (copy, distribute, transmit) and to adapt it, under the condition that the authors are given credit and that in the event of reuse or distribution, the terms of this license are made clear.